In Chicago, Jane Thompson, manager at a healthcare staffing company, was faced with a daunting challenge. Her team had received an overwhelming number of healthcare resumes for critical positions.
While they had moved on from the manual process of sifting through stacks of resumes, extracting information, and matching qualifications, the resume parsing solution they were using also left a lot to be desired. It was generic, prone to errors, and above all, there was no facility to run specific queries. Resume retrieval and scoring was still a manual process.
Jane’s experience mirrored that of many healthcare staffing professionals across the nation. They struggled to find the best candidates quickly, risking delays in patient care. Little did Jane know that a transformative solution was on the horizon – one that would use AI and NLP to revolutionize resume processing in healthcare staffing.
In this article, we’ll explore the challenges Jane faced and how AI-powered resume parsing is reshaping healthcare recruitment, making it efficient and precise.
Challenges in Healthcare Staffing Resume Processing
We know that recruiters in the healthcare staffing industry face a formidable challenge when it comes to resume processing. The very essence of their work, which involves matching skilled healthcare professionals with critical positions, hinges on the ability to assess candidate qualifications swiftly and accurately. Let us look at the current methods and practices that present significant hurdles.-
Overwhelming Volume of Resumes:
-
Time-Consuming Manual Review:
-
Half-baked Resume Parsing Solutions:
-
Risk of Missing the Perfect Candidate:
-
Need for Efficient and Precise Matching:
A Definitive Solution
The urgency and complexity of healthcare staffing demands a solution that can streamline the process, reduce the burden on recruiters, and ensure that no qualified candidate goes unnoticed.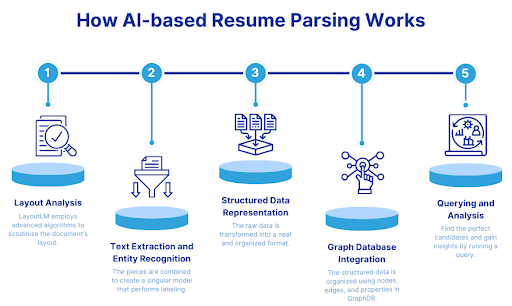
Step 1: Layout Analysis:
Layout analysis is where it all begins. Resumes come in various formats, and LayoutLM (by HuggingFace) employs advanced algorithms to scrutinize the document’s layout, precisely identifying distinct sections. It’s akin to having an expert eye that can pinpoint the exact location of each piece of crucial data, even if it’s hidden within a complex layout. LayoutLM’s ability to comprehend the document’s layout ensures that no valuable information is missed.Step 2: – Text Extraction and Entity Recognition:
LayoutLM then combines the pieces to create a singular model that performs labeling using positional, text-based, and image information. In a healthcare resume, these sections typically include personal details, educational qualifications, work experience, skills, certifications, and professional affiliations.Step 3: Structured Data Representation:
Next, the raw data is transformed into a neat and organized format. It’s like sorting puzzle pieces to create a clear picture.Organizing the Information:
- Categorizing: We group information into categories like personal details, education, work history, skills, certifications, and affiliations. Each piece of info gets a label that makes it easy to find later.
- Making Things Uniform: Sometimes, data appears in different ways. For example, job titles might be listed as “Registered Nurse” or just “RN.” We make all this data consistent so it’s easier to search and compare.
- Connecting the Dots: Some details relate to each other. For example, education is linked to work experience, and certifications are connected to skills. We identify these connections to build a more complete picture of each candidate.
Mapping to Healthcare Requirements:
One crucial part is mapping this organized data to a blueprint made specifically for healthcare staffing. Here’s why it matters:- Made for Healthcare: This blueprint understands the unique needs of healthcare staffing. It ensures that the data matches exactly what the industry requires.
- Quick Retrieval: When recruiters need specific info, the system can quickly find it using this blueprint. It’s like having a map to locate skills within a huge library of resumes.
- Keeping Things Right: The blueprint enforces rules to ensure data is accurate and follows industry standards. This helps with matching candidates and staying compliant.
Step 4: Graph Database Integration:
In this step, we organize the structured data using GraphDB. To understand GraphDB, think of a digital map with points and lines connecting them. Each point represents a specific detail from a resume, like a candidate’s name or a certification. The lines connect these pieces to show how they relate to each other, like connecting a candidate to their education or work history.How It Works:
- Nodes: Each node holds a specific piece of information.
- Edges: They show how different pieces of information are linked.
- Properties: These are like labels that give extra info about nodes and edges.
How this Helps:
- Find related information fast: If we’re interested in a candidate’s education, we can easily follow the lines to their certifications or work history.
- Do complex searches: For example, we can look for candidates with specific certifications who’ve worked in particular places.
- Easily update information: If something changes, we can adjust the map without upsetting anything.
Step 5: – Querying and Analysis:
Finally, you use your data to find the perfect candidates quickly and to gain valuable insights. You instantly locate the exact resume details you’re looking for by running a query. This makes finding the right candidates incredibly fast and accurate. For example:- Qualifications: If you need candidates with a Master’s degree in Nursing, the system can find them for you in no time.
- Skills: Looking for nurses with specific skills like “Critical Care”? The system can find them quickly.
- Certifications: Need candidates with certifications like “BLS” or “ACLS”? It can pull them up fast.
- Experience: If you want candidates with at least five years of ICU experience, the system can filter out those who fit the bill.
Accelerating Efficiency and Multiplying Business Impact
The integration of AI and NLP in healthcare staffing is bringing about significant business benefits. Blackstraw’s Clients Have Reported:- 5X Better Job Matching: The accuracy of candidate matches is greatly improving, thanks to algorithms that analyze job descriptions and align them with candidate profiles, ensuring that healthcare facilities receive the talent they require.
- Reduced Offer Wait Time To < 30 Mins: Recruiters are easily handling high volumes of resumes, regardless of the scale, ensuring that no potential candidate is overlooked.
- Slashed credentialing time by 60%: This approach not only accelerates the recruitment process but also significantly enhances the overall efficiency of the healthcare staffing operation.
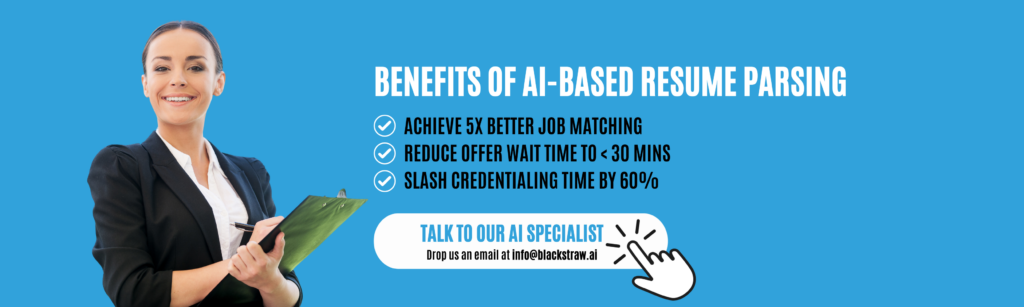
Conclusion
AI and NLP-based resume parsing is transforming the healthcare staffing industry, reducing recruiter workload, enhancing candidate matching accuracy, and streamlining operations. The profound business impact of this innovation, including time savings and improved client outcomes, cannot be overstated. As healthcare staffing becomes increasingly dynamic and competitive, it is paramount that organizations consider implementing AI-driven solutions in their recruitment processes. Embracing technology boosts efficiency and precision and ensures that healthcare facilities can swiftly secure the right talent to provide quality care.Ready to explore the possibilities of AI
for your business?
Talk to Us
for your business?