Reasons Why You Need MLOps
Streamline your Machine Learning projects with Blackstraw MLOps as a service
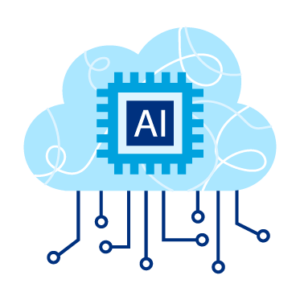
Did you know that only 1 out of 10 data science projects end up delivering value to organizations as 90 percent of machine learning models fail to make it to production. This is due to the ever-evolving data, which requires continuous monitoring, training, and redeployments. As a result, bringing ML models to production can be challenging and time-consuming process that requires the discipline of delivering models through repeatable and efficient workflows known as machine learning operations (MLOps).
With Blackstraw, you can avoid the expense and effort for developing and maintaining your Machine Learning models. Our team of data experts has developed proprietary MLOps frameworks and approaches, which can be tailored to fit seamlessly into your existing ecosystem; allowing you to reduce the time-to-deployment, minimize risks and improve operational performance.
Business Benefits
Accelerated Innovation & Growth
Faster time to deployment and iteration of ML models results in a shorter time to market, allowing businesses to innovate and grow at a faster pace.
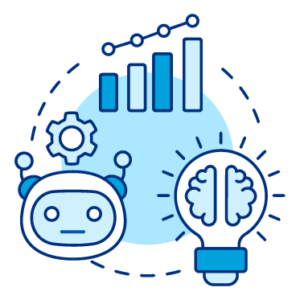
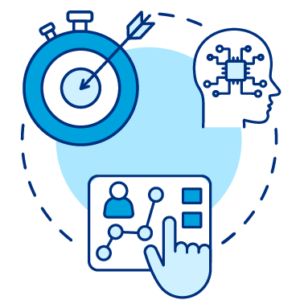
Boost Business Decisions with Reliable Model Reproducibility
Achieve greater transparency, reliability, and accountability in machine learning processes through model reproducibility.
Efficient Management of Data and Model Drift
Quickly monitor and manage data and model drift for operationalized models ensures that models remain relevant and performant, resulting in optimized business outcomes.
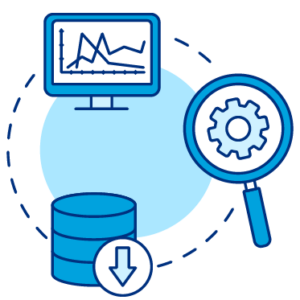
Blackstraw’s MLOps as a service offerings
Our Technology Expertise
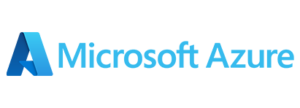
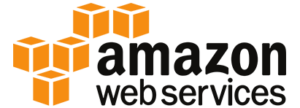
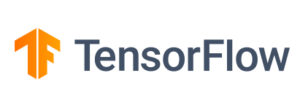
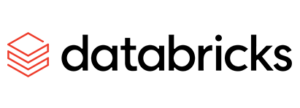
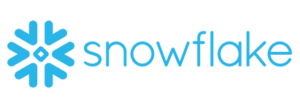
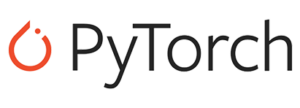
Why Blackstraw
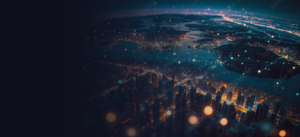
Don’t let the challenges of
machine learning
management slow down your
innovation and growth
A diversified industrial manufacturer
Detect and track suspicious people, cars and objects. Read More
American information, data and measurement firm
Deep-learning based solution supplemented with Hand-crafted features and OCR. Read More
Leading integrated inventory services company
AI-led predictive model to predict enrollment and attendance for a posted job (gig). Read More