The capabilities of large language models (LLMs) have experienced remarkable advancements in recent years. These sophisticated AI-driven tools, functioning as deep learning artificial neural networks, have undergone substantial refinement in their abilities. Through extensive training on vast datasets, LLMs have evolved to effectively leverage billions of parameters, enabling them to excel in various natural language processing (NLP) tasks.
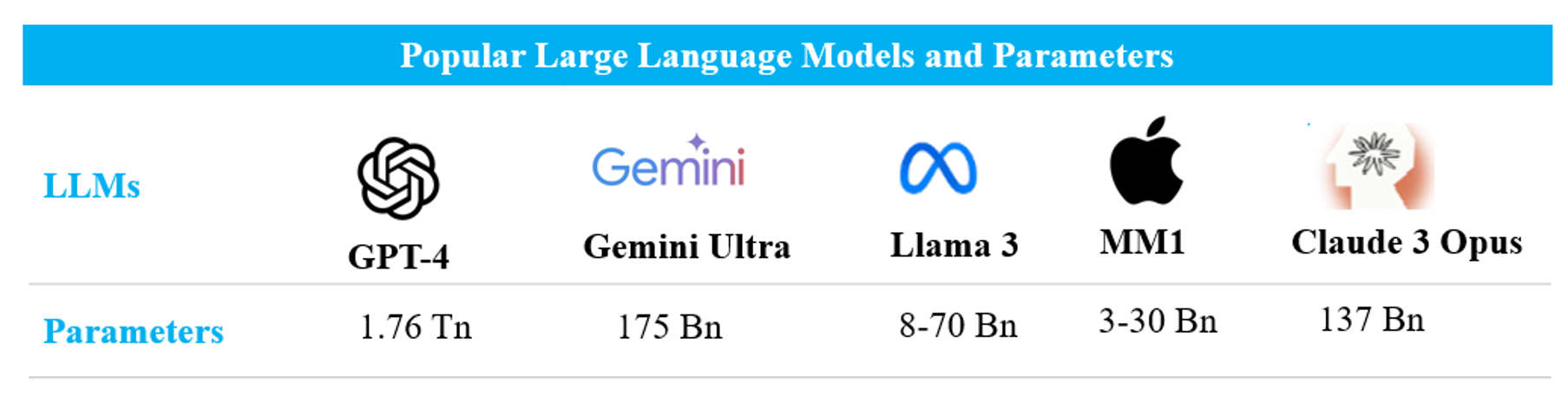
Nonetheless, LLMs come with certain drawbacks. A significant challenge lies in their high cost for training and operation, owing to their demand for substantial computing power. Moreover, LLMs may occasionally produce biased or offensive text, presenting ethical concerns. The large dataset they’ve trained also increases risks of inaccuracy or unintended behavior. Consequently, there is an emerging interest in Small Language Models (SLM)s. While not as potent as LLMs, SLMs offer cost-efficiency in training and operation. Furthermore, they are less prone to generating biased or offensive content, addressing ethical considerations more effectively.
Overview of Small Language Models (SLM)
Small language models are scaled-down versions of larger AI models like GPT-4 or Gemini Ultra. They are designed to perform similar tasks—understanding and generating human-like text—but with fewer parameters. Subsequently, they are cheaper and more environmentally responsible. Their more limited scope and data make them better suited and customizable for focused business use cases like chat, text search/analytics, and targeted content generation. Here are several significant features contributing to the increasing popularity of small language models.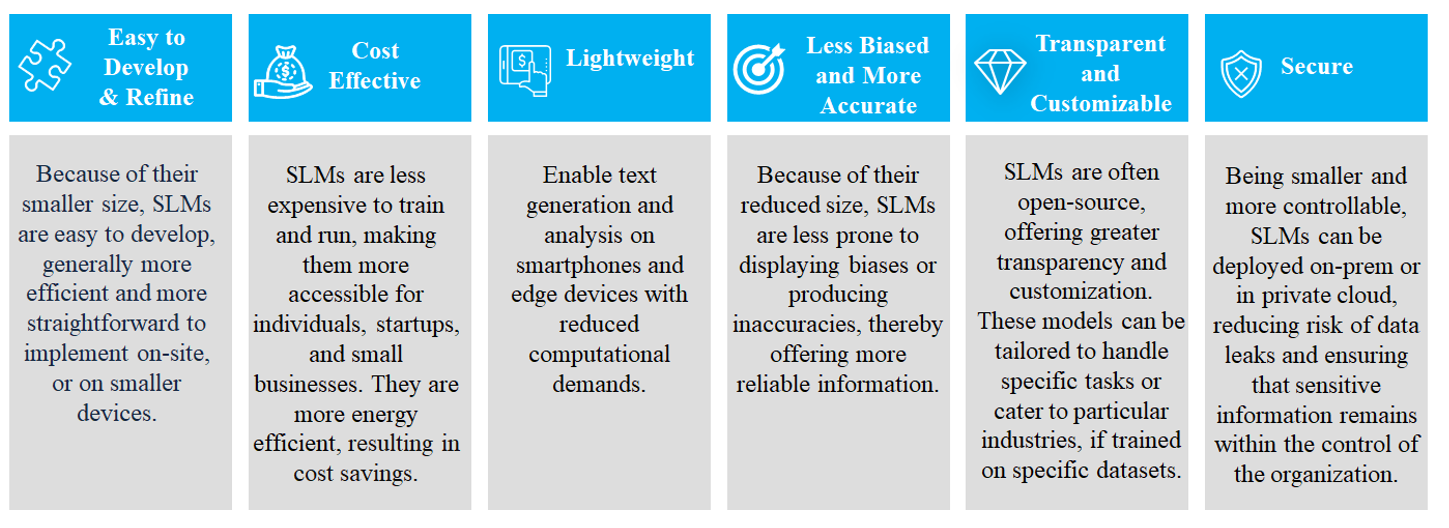
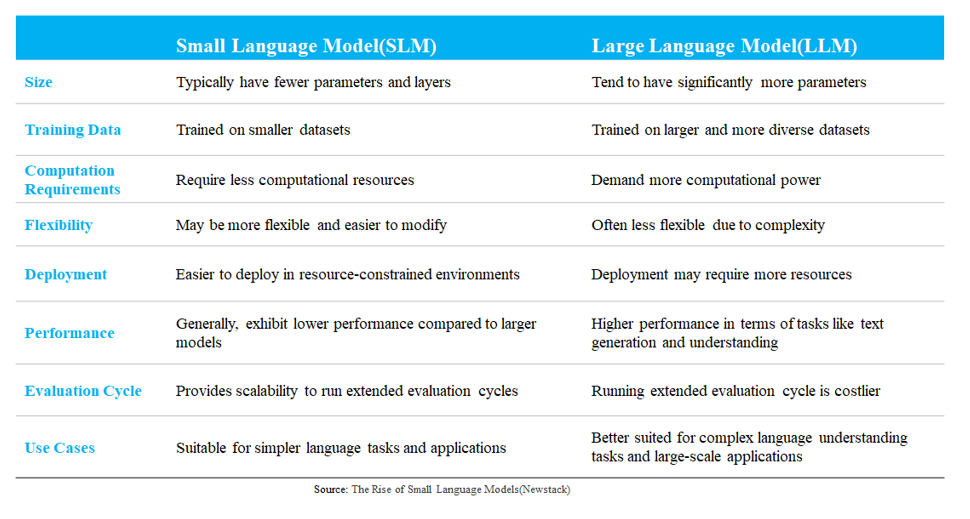
Market Size and Outlook
The market for SLMs, is poised for significant growth, driven by their rising popularity. According to Valuate Research, the global market for SLMs was valued at $5.9 billion in 2023 and is projected to soar to $16.9 billion by 2029, reflecting a robust CAGR of 18% during this period.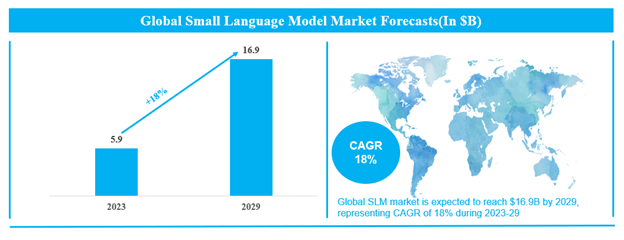
Application of Small Language Models (SLM)
SLMs can generate high-quality output, especially in specific domains where they have been fine-tuned. For example, SLMs’ compact size and efficient computation make them well-suited for deployment on edge devices, mobile applications, and resource-constrained environments as well., Apple has recently released eight small AI language models intended for use on smartphones and other devices as proof-of-concept research releases, made available under Apple’s open source-like Sample Code License. In general, these models can be tuned in domains such as:- Sentiment Analysis: Analyzing sentiment in text—whether it’s positive, negative, or neutral. SLMs help businesses gauge customer feedback, monitor social media sentiment, and improve user experiences.
- Named Entity Recognition: SLMs work exceptionally well on descriptive and abbreviated data records and accurately extract customized entity classes with few-shot training. Delivers low-latency and high accurate results.
- Content Classification: Customized content classification of language content with 100s can be achieved through few-shot or QLoRa fine-tuning approaches.
- Personalized Recommendations: SLMs can tailor recommendations based on user preferences. Whether it’s suggesting movies, books, or products, SLMs enhance personalized content delivery.
- Low-Resource Language Processing: In languages with limited training data, SLMs provide a viable solution. They enable NLP tasks even when resources are scarce.
- Text Classification and Information Retrieval: SLMs classify text into predefined categories (e.g., spam detection, topic labeling).They also enhance search engines by improving document retrieval.
- Language Translation: SLMs can be fine-tuned for specific language pairs, making them suitable for low-resource language translation. They offer an efficient alternative to large-scale translation models.
Embark on Your SLM Journey with Blackstraw
At Blackstraw, we recognize the transformative potential of SLMs and are committed to assisting businesses in unlocking their full capabilities. Drawing on our expertise in AI, specifically in NLP, computer vision, and machine learning, we have empowered numerous clients through a range of applications including text generation, summarization, language translation, sentiment analysis, text classification, and information retrieval across diverse industries. We have utilized our proficiency in customizing SLMs to support our global customers, in various ways including:- Customized classification of language content, with hundreds of labels, which enables our customers in product category identification across e-commerce and store-based data, while intent identification facilitates enhanced automation efficiency in incident management, among other benefits.
- Retail product attribute enrichment, semantic similarity search within enterprise content and recruitment optimization through job matching solutions, through recommendation and similarity search use cases, trained for vectorization tasks, demonstrating cutting-edge efficiency.
- Taxonomy mapping, third-party data harmonization, manufacturing to retail systems mapping, fields extraction from forms, invoices, emails, in numerous data enrichment and harmonization applications.
for your business?